XIX Simposio Internacional de Ingeniería Eléctrica
SIE 2021
Resumen
Mamografía es una técnica reconocida para el diagnóstico precoz de las lesiones benignas o malignas en las mamas. En las imágenes mamográficas el contraste es muy bajo y el ruido puede enmascarar algún hallazgo. El objetivo de este trabajo fue estudiar la reducción del ruido gaussiano en una imagen mamográfica.
Se partió de una imagen ida de la base mini MIAS y se utilizó el sofware MATLAB 2017A. Se mejoró el contraste y se recortó la parte con lesiones. Se incorporó ruido gaussiano (media 0 y variancia variando entre 1E-3 y 1E-1) y se utilizaron filtros adaptativos de 2D: LMS, LMS normalizado (NLMS) y LMS-Newton (LMSN). El número de realizaciones (N) fue igual a 100, el tamaño del kernel fue 4x4 y se varió el factor de convergencia (µ) entre 1E-6 y 3E-4. Para el NLMS, el valor de gama varió entre 10-8 y 10-14, mientras que para el algoritmo LMSN el valor de alfa varió entre 6E-4 y 6E-6 y gama varió entre 1E-3 y 1E-1.
Las mejores imágenes filtradas para LMS fueron para ruido gaussiano de variancia 1E-2, para µ = 1E-6 obteniendo MSE 0.0130 y tiempo de corrida de 1.5873E3segundos. Para NMLS con ruido de variancia 1E-2 las mejores imágenes fueron para α=1E-12,µ = 3E-6, MSE 0.0027 y 1.5301E3 segundos. Para NLMSN ruido de variancia 1E-2, α=6E-4, γ=1E-1, µ=3E-6, MSE 0.0191 y 3.5765E3 segundos. Se concluye que los algoritmos anteriores podrían utilizarse para reducir el ruido gaussiano de una imagen mamográfica, a partir de la imagen deseada.
Abstract
Mammography is a recognized technique for the early diagnosis of benign or malignant breast lesions. In mammographic images, the contrast is very low and noise can mask a finding. The objective of this work was to study the reduction of Gaussian noise in a mammographic image.
It was started from an image of the mini MIAS base and the MATLAB 2017A software was used. The contrast was improved and the part with lesions was cut out. Gaussian noise was incorporated (mean 0 and variance varying between 1E-3 and 1E-1) and adaptive 2D filters were used: LMS, normalized LMS (NLMS) and LMS-Newton (LMSN). The number of realizations (N) was equal to 100, the kernel size was 4x4 and the convergence factor (µ) was varied between 1E-6 and 3E-4. For the NLMS, the range value varied between 10-8 and 10-14, while for the LMSN algorithm the alpha value varied between 6E-4 and 6E-6 and range varied between 1E-3 and 1E-1.
The best filtered images for LMS were for Gaussian noise of variance 1E-2, for µ = 1E-6 obtaining MSE 0.0130 and run time of 1.5873E3 seconds. For NMLS with 1E-2 variance noise the best images were for α = 1E-12, µ = 3E-6, MSE 0.0027 and 1.5301E3 seconds. For NLMSN noise variance 1E-2, α = 6E-4, γ = 1E-1, µ = 3E-6, MSE 0.0191 and 3.5765E3 seconds. It is concluded that the previous algorithms could be used to reduce the Gaussian noise of a mammographic image, starting from the desired image.
Sobre el ponente
MsC. Rosana Pirchio
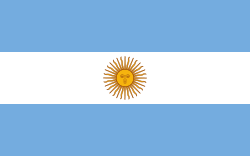