XX International Symposium of Electrical Engineering
SIE 2023
Abstract
Creating algorithms and systems that can process and store large amounts of data represents a great scientific, economic, and practical challenge. The application of machine learning (ML) to these problems is not trivial, and even less so if the processing of these algorithms needs to be distributed to handle the large computational load of data analysis and decision making. This paper presents a distributed and robust architecture to train, deploy, and execute distributed failure detection algorithm pipelines improving their Robustness and Precision. The solution is based on Smart Operational Realtime Bigdata Analytics (SORBA), a patented distributed architecture. The architecture combines the metrics of Robustness and Precision to automatically optimize the selection of industrial failure detection machine learning algorithm pipelines and their hyperparameters. A system of modules is developed for the acquisition, normalization, data conditioning, training, deployment, and online execution of machine learning algorithm pipelines. The solution was validated by comparing the Machine Learning (ML) results of three use cases: an industrial motor, a wastewater blower, and a locomotive battery, with those achieved with Spark. The experiments showed an average improvement on the Precision Score of 28.76% and Robustness Index of 10.9%. The solution streamlines the implementation of successful applications and improves the performance of these indicators with respect to the solutions currently available in the Spark MLlib.
Resumen
Crear algoritmos y sistemas que puedan procesar y almacenar grandes cantidades de datos representa un gran desafío científico, económico y práctico. La aplicación del aprendizaje automático (ML) a estos problemas no es baladí, y menos aún si es necesario distribuir el procesamiento de estos algoritmos para manejar la gran carga computacional del análisis de datos y la toma de decisiones. Este artículo presenta una arquitectura distribuida y robusta para entrenar, implementar y ejecutar canales de algoritmos distribuidos de detección de fallas mejorando su robustez y precisión. La solución se basa en Smart Operational Realtime Bigdata Analytics (SORBA), una arquitectura distribuida patentada. La arquitectura combina las métricas de robustez y precisión para optimizar automáticamente la selección de canales de algoritmos de aprendizaje automático de detección de fallas industriales y sus hiperparámetros. Se desarrolla un sistema de módulos para la adquisición, normalización, acondicionamiento de datos, capacitación, implementación y ejecución en línea de canales de algoritmos de aprendizaje automático. La solución se validó comparando los resultados del aprendizaje automático (ML) de tres casos de uso: un motor industrial, un soplador de aguas residuales y una batería de locomotora, con los logrados con Spark. Los experimentos mostraron una mejora promedio en la puntuación de precisión del 28,76 % y el índice de robustez del 10,9 %. La solución agiliza la implementación de aplicaciones exitosas y mejora el rendimiento de estos indicadores con respecto a las soluciones actualmente disponibles en Spark MLlib.
About The Speaker
Ing. Yandy Perez
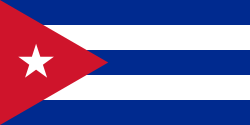
Discussion