10th International Conference of Mechanical Engineering
COMEC 2019
Abstract
The recognition of logistics objects is an essential prerequisite for the optimization of operational logistics processes and can be performed among others via image-based methods. However, the lack of available data for training domain-specific recognition models remains a practical problem. For this reason, we present an approach to solving this problem. The core principle of our approach is the automated generation of image data from 3D models, in which the appearance of the objects varies through variations of different parameters. The first results are promising: Without any real image data, we have created a neural network for recognition of real objects with a recall quality of 86%.
Resumen
The recognition of logistics objects is an essential prerequisite for the optimization of operational logistics processes and can be performed among others via image-based methods. However, the lack of available data for training domain-specific recognition models remains a practical problem. For this reason, we present an approach to solving this problem. The core principle of our approach is the automated generation of image data from 3D models, in which the appearance of the objects varies through variations of different parameters. The first results are promising: Without any real image data, we have created a neural network for recognition of real objects with a recall quality of 86%.
About The Speaker
Hagen Borstell
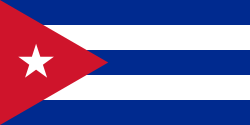