7th International Symposium of Pharmaceutical Sciences
VII SICF
Abstract
Leishmaniasis is poverty-related disease endemic in almost 100 countries worldwide, with morbidity and mortality growing daily. Currently used drugs exhibit several disadvantages including toxicity, high costs and route of administration. Consequently, the development of new treatments for leishmaniasis is a priority in the field of neglected tropical diseases. The aim of this work is to develop computational models to identify new chemical compounds with potential anti-leishmanial activity. A data set of 116 organic chemicals, assayed against promastigotes of Leishmania amazonensis, is used to develop the theoretical models. For this study, we employed Dragon software to calculate the molecular descriptors and WEKA software to obtain the machine learning (ML) models. The ML models showed accuracy values between 82% and 91%, for training set. The models developed with k-nearest neighbours and classification trees showed sensitivity values of 97% and 100%, respectively; while the models developed with artificial neural networks and support vector machine showed specificity values of 94% and 92%, respectively. With the aim of validate our models we use an external test-set, with good behavior for all models. A virtual screening was performed and about 150 compounds were identified as potential anti-leishmanial by all the ML models. This research remarks the merits of ML-based techniques as an alternative to traditional methods to find new chemical compounds with anti-leishmanial activity.
Resumen
Leishmaniasis is poverty-related disease endemic in almost 100 countries worldwide, with morbidity and mortality growing daily. Currently used drugs exhibit several disadvantages including toxicity, high costs and route of administration. Consequently, the development of new treatments for leishmaniasis is a priority in the field of neglected tropical diseases. The aim of this work is to develop computational models to identify new chemical compounds with potential anti-leishmanial activity. A data set of 116 organic chemicals, assayed against promastigotes of Leishmania amazonensis, is used to develop the theoretical models. For this study, we employed Dragon software to calculate the molecular descriptors and WEKA software to obtain the machine learning (ML) models. The ML models showed accuracy values between 82% and 91%, for training set. The models developed with k-nearest neighbours and classification trees showed sensitivity values of 97% and 100%, respectively; while the models developed with artificial neural networks and support vector machine showed specificity values of 94% and 92%, respectively. With the aim of validate our models we use an external test-set, with good behavior for all models. A virtual screening was performed and about 150 compounds were identified as potential anti-leishmanial by all the ML models. This research remarks the merits of ML-based techniques as an alternative to traditional methods to find new chemical compounds with anti-leishmanial activity.
About The Speaker
Juan Alberto Castillo Garit
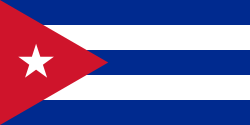