11nd International Conference of Mechanical Engineering
COMEC2023
VI Symposium of Logistics and Quality Management
Abstract
Demand forecasting is one of the key points of planning. In this sense, many companies have sought ways to apply innovative methods to obtain future trends in the demand studied. On the other hand, with the increase in computing capabilities in recent years, there has been a boom in the use of neural networks in demand prediction. The objective of this work is to propose a methodological tool to make forecasts using machine learning algorithms. In this case, a multilayer Perceptron neural network was used to forecast hotel demand for the City and Resort hotels in the Santa María de Villa Clara keys. The model gave a better result in the forecast of the demand in the Hotel Resort where a MAE=68.72, RMSE=80.12 and MAPE=7.41% was obtained. The model proved to be able to reliably predict the demand trend for the Resort hotel, yielding values very close to the real ones. In the case of the Ciudad hotel, more data is needed to obtain an acceptable result in the demand forecast.
Resumen
La predicción de la demanda constituye uno de los puntos clave de la planificación. En este sentido, muchas empresas han buscado la forma de aplicar métodos novedosos para obtener las tendencias futuras de la demanda estudiada. Por otro lado, con el aumento de las capacidades de cómputo en los últimos años, se ha evidenciado un auge en la utilización de redes neuronales en la predicción de la demanda. El presente trabajo tiene como objetivo proponer una herramienta metodológica para realizar pronósticos utilizando algoritmos de aprendizaje de máquinas. En este caso, se utilizó una red neuronal Perceptrón multicapa para pronosticar la demanda hotelera de los hoteles Ciudad y Resort en los cayos Santa María de Villa Clara. El modelo arrojó un mejor resultado en el pronóstico de la demanda en el Hotel Resort donde se obtuvo un MAE=68.72, RMSE=80.12 y MAPE=7.41%. El modelo demostró ser capaz de predecir de manera fiable la tendencia de la demanda del hotel Resort, arrojando valores muy cercanos a los reales. En el caso del hotel Ciudad, se necesitan más datos para obtener un resultado aceptable en la predicción de la demanda.
About The Speaker
Andrey Vinajera Zamora
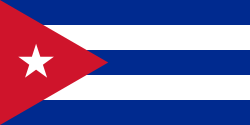
Discussion