Executive Secretary
19th International Symposium of Electrical Engineering
SIE 2021
Abstract
The present work shows the design of a three-dimensional tracking filter for maneuvering targets designed with the help of the Sensor Fusion and Tracking Toolbox™ present in Matlab 2020a. As a method of estimating the three-dimensional components of position, velocity and acceleration of each target, a bank of extended Kalman filters is used in order to reduce the errors introduced in the observation and the estimation of the state variables not measured directly. Constant speed, constant acceleration and constant rotation models are implemented in the filter bank. The conformation of the final estimate is achieved with an interactive multiple model (IMM) algorithm, which is responsible for combining the state hypotheses of the multiple models present in the filter bank, to obtain a better estimate of the state of a maneuvering target. The operation of the IMM algorithm is determined by a Markov chain, in charge of controlling how the commutation occurs between the motion models. In order to evaluate the filter´s performance, 50 trajectories with 900m/s speed and maneuvers in the 3 axes were generated with noise and without it. As a result, it was obtained that the estimation of said trajectories showed high correspondence between the real trajectory and the one estimated for both cases.
Resumen
El presente trabajo muestra el diseño de un filtro de seguimiento tridimensional para objetivos maniobrantes diseñado con la ayuda del Sensor Fusion and Tracking Toolbox™ presente en Matlab 2020a. Se emplea como método de estimación de las componentes tridimensionales de posición, velocidad y aceleración de cada objetivo, un banco de filtros Kalman extendidos con el objetivo de reducir los errores introducidos en la observación y la estimación de las variables de estado no medidas directamente. En el banco de filtros se implementan modelos de velocidad constante, aceleración constante y giro constante. La conformación de la estimación final se logra con un algoritmo de modelo múltiple interactivo (IMM), el cual se encarga de la combinación de hipótesis de estado de los múltiples modelos presentes en el banco de filtros, para obtener una mejor estimación del estado de un objetivo maniobrante. El funcionamiento del algoritmo IMM está determinado por una cadena de Markov, encargada de controlar cómo ocurre la conmutación entre los modelos de movimiento Para comprobar el funcionamiento de este filtro, se generaron 50 trayectorias con velocidad de 900m/s y maniobras por los tres ejes en presencia de ruidos y sin este. Como resultado se obtuvo que la estimación de dichas trayectorias presentó elevada correspondencia entre la trayectoria real y la estimada para ambos casos.
About The Speaker
José Ernesto Vargas Fernández
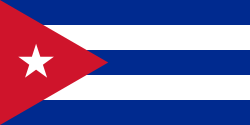