Executive Secretary
19th International Symposium of Electrical Engineering
SIE 2021
Abstract
• Problem: Due to the COVID-19 pandemic, computer vision researchers have worked on the automatic identification of this disease using radiological images. The results obtained by the automatic classification methods far exceed those of human specialists, having even registered a sensitivity of 100%. However, prestigious radiology societies have stated that the use of this type of images alone is not recommended as a diagnostic method. According to radiologists, the patterns they present in these images are nonspecific and subtle, overlapping with other viral pneumonias
• Objective(s): This research aims to elucidate from the analysis of explainable artificial intelligence techniques the robustness and level of generalization of computer vision methods proposed to identify COVID-19 using chest X-ray images. Also, to alert researchers and reviewers to the problem of shortcut learning.
• Methodology: In this study, recommendations are followed to identify whether COVID-19 automatic classification models are affected by shortcut learning. For this purpose, articles using explainable artificial intelligence methods are reviewed.
• Results and Discussion: It was evidenced that when using the full chest X-ray image or the lung bounding box, the image regions that contribute most to the classification appear outside the lung region, something that does not make sense.
• Conclusions: The results indicate that, so far, existing models present the problem of shortcut learning, which makes them unsuitable for use in clinical settings.
Resumen
• Problemática: Con motivo de la pandemia de COVID-19, los investigadores en visión por computadora han trabajado en la identificación automática de esta enfermedad usando imágenes radiológicas. Los resultados obtenidos por los métodos de clasificación automática superan con creces a los especialistas humanos, habiéndose registrado incluso una sensibilidad del 100%. Sin embargo, prestigiosas sociedades de radiología han manifestado que no se recomienda el uso de este tipo de imágenes por sí solas como método de diagnóstico. Según los radiólogos, los patrones que presentan en estas imágenes son inespecíficos y sutiles, solapándose con otras neumonías virales.
• Objetivo(s): Esta investigación pretende dilucidar a partir del análisis de técnicas de inteligencia artificial explicables la robustez y el nivel de generalización de los métodos de visión por computadora propuestos para identificar COVID-19 utilizando imágenes de radiografías de tórax. Asimismo, alertar a los investigadores y revisores sobre el problema del aprendizaje por atajos.
• Metodología: En este estudio se siguen recomendaciones para identificar si los modelos de clasificación automática de COVID-19 se ven afectados por el aprendizaje por atajos. Para ello, se revisan los artículos que utilizan métodos de inteligencia artificial explicable en dicha tarea.
• Resultados y discusión: Se evidenció que al utilizar la imagen de radiografía de tórax completa o el cuadro delimitador de los pulmones, las regiones de la imagen que más contribuyen a la clasificación aparecen fuera de la región pulmonar, algo que no tiene sentido.
• Conclusiones: Los resultados indican que, hasta ahora, los modelos existentes presentan el problema de aprendizaje por atajos, lo cual los hace inapropiados para ser usados en entornos clínicos.
About The Speaker
José Daniel López Cabrera
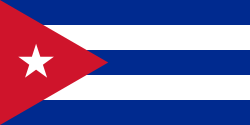