Simposio Internacional de Ciencias Farmaceúticas
SICF
Abstract
In this study, we present a KNIME automated workflow to modeling a large, diverse, and highly imbalanced dataset of compounds with antileishmanial activity. Because the data is strongly biased toward inactive compounds, a novel strategy was implemented based on the selection of different balanced training sets and a further consensus model using single decision trees as the base model and three criteria for output combinations. The decision tree consensus was adopted after comparing its classification performance to consensuses built upon Gaussian-Naïve-Bayes, Support Vector-Machine, Random-Forest, Gradient-Boost, and Multi-Layer-Perceptron base models. All these consensuses were rigorously validated using internal and external test validation sets and were compared against each other using Friedman and Bonferroni−Dunn statistics. For the retained decision tree-based consensus model, which covers 100% of the chemical space of the dataset and with the lowest consensus level, the overall accuracy statistics for test and external sets were between 71 and 74% and 71 and 76%, respectively, while for a reduced chemical space (21%) and with an incremental consensus level, the accuracy statistics were substantially improved with values for the test and external sets between 86 and 92% and 88 and 92%, respectively. An external test set II made of 139 active structures with confirmed antileishmanial EC50 activity from the PubChem AID 2008 dataset and a list of 10 commercially available compounds served to evaluate our methodology at classifying and ranking the activity of these confirmed active compounds and commercially available drugs, showing its potential interest for testing further commercially available libraries or marketed drugs with the aim of repurposing. The open-access workflow provided with this study can directly be used for this task.
Resumen
Las predicciones in-silico de la actividad antileishmanial usando modelos de Relaciones Cuantitativas Estructura-Actividad (QSAR) han sido desarrollados sobre bases de datos pequeñas y limitadas a series congenéricas de compuestos. La actual disponibilidad de grandes y diversas bases de datos obtenidas de High-Throughput Screening (HTS) provee la oportunidad a la comunidad científica de modelar esta actividad. En este estudio, se presenta un flujo de trabajo implementado en KNIME que permite modelar grandes, diversas y altamente desbalanceadas bases de compuestos. La estrategia se basa en la selección de diferentes series de entrenamiento balanceadas que permiten la construcción de un modelo consenso basado en árboles de decisión. El consenso basado en árboles fue elegido después de una comparación de su desempeño con consensos que usaron Gaussian-Naïve-Bayes, Support Vector-Machine, Random-Forest, Gradient-Boost, and Multi-Layer-Perceptron como modelos base. Los modelos fueron rigurosamente validados usando series de prueba y externa y fueron comparados entre ellos usando los estadísticos de Friedman and Bonferroni−Dunn. Para el modelo consenso elegido, el nivel de consenso más bajo que cubre el 100% del espacio químico, las estadísticas de predicción están entre 71 y 74% (prueba) y 71 y 76% (externo), mientras que para un espacio químico reducido hasta el 21% (después de usar un nivel de consenso incremental), las estadísticas ascienden hasta el 92% (ambos conjuntos). Un conjunto externo II conformado por 139 estructuras activas con actividad antileishmanial (EC50) reportada en la base de datos PubChem AID 2008, junto a 10 compuestos comerciales se utilizaron para evaluar nuestra metodología y clasificar y ranquear la actividad de esas estructuras activas. Los resultados mostraron el potencial de esta herramienta en el tamizaje virtual de librerías de compuestos así como en el reposicionamiento de fármacos. El flujo de trabajo presentado aquí permite a otros investigadores reproducir nuestro diseño y usarlo en sus propios problemas de QSAR que utilicen bases de datos altamente desbalanceadas.
About The Speaker
Ph. D. Aliuska Morales Helguera
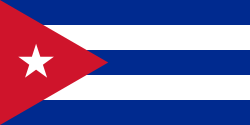